Companies looking to the future
07-Dec-2023 - Last updated on 07-Dec-2023 at 16:19 GMT Phase 1 Clinical Trial
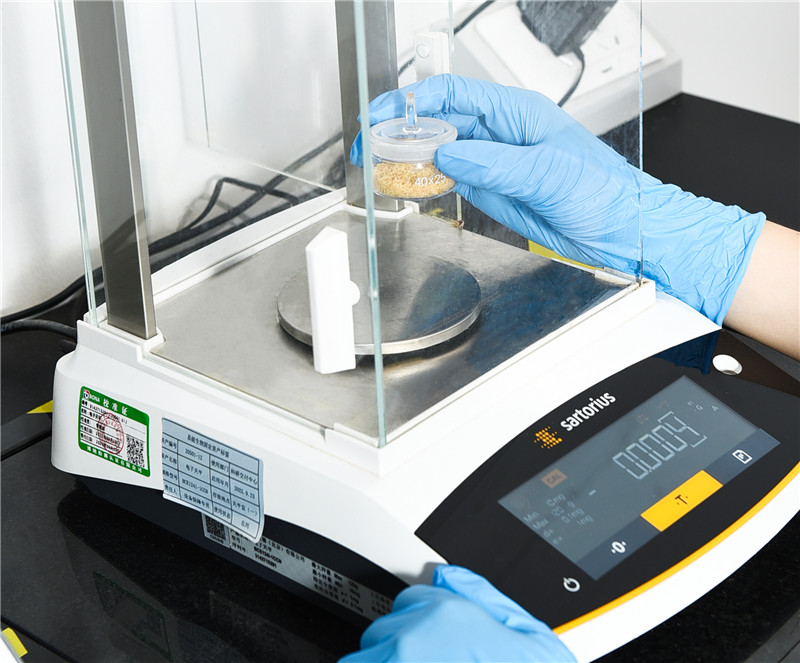
Related tags Marine derived ingredients trends Formulation
According to a report from global market research firm Skyquest Technologies, "the global marine-based skin care ingredient market is projected to reach $959.86 billion by 2030," with a compound annual growth rate (CAGR) of 6.6% in the forecast period of 2023-2030. As CosmeticsDesign has previously reported, marine ingredients in cosmetics and personal care were one of the biggest trends in 2023, including formulation components like algae, salt water, and seaweed.
One brand that has embraced ocean-inspired ingredients is Laguna Herbals, a California-based natural and organic skincare and body product company. The indie brand's product collection includes lip, skin, and body care products and a recently launched baby care line. To learn more about Laguna Herbals' product formulation process, including ingredient development, challenges that need to be addressed before release, and plans for future product launches, CosmeticsDesign interviewed Andrea Sands, founder of Laguna Herbals, for her insights.
AS: I have always believed in natural alternatives, and my interest in organic and natural skincare came from my interest in alternative medicine. I have studied herbs' healing actions on your health for years and have overcome some minor yet chronic health issues by seeking alternatives when traditional medicine didn't work.
I decided to become a certified herbalist and studied with some of the most renowned herbalists in the country. Through my studies and coursework, I came up with a love of the healing topical applications of balms and oils infused with herbal actives that have been used for centuries.
Everything you ingest or put on your skin has either a positive or negative impact on your health. We are creatures of habit, so when we repeatedly use the same products daily – harsh ingredients can bioaccumulate in our system and negatively impact our health.
This is the danger of using products that have harsh and even toxic chemicals that can bioaccumulate in your system and cause a range of adverse health issues.
AS: Being a beach city brand that is based in Laguna Beach, CA., it seemed only natural that we would create products infused with ingredients from the ocean, and these products incorporate both ocean algae and giant sea kelp. At first, we were just looking to add a jelly mask. Once we got to work with these new ingredients, we immediately decided to make a whole collection of ocean-inspired products to form a complete face routine featuring our Ocean Pure Cleanser, Ocean Jelly Mask, Ocean Glow Drops (hyaluronic acid serum), and Ocean Milk moisturizer.
All products in the collection use effective, scientific, and trendy ingredients of niacinamide, hyaluronic acid, ocean algae, sea kelp, and blue tansy oil. We formulate all our products from scratch: we don't use premade cosmetic bases because we must have complete control over our raw ingredient sourcing and production process.
This collection took a lot of research and testing to ensure we were using the most effective yet natural ingredients with a sound shelf life.
AS: There are many natural brands out there, but what sets us apart are the points below.
The term "herbalist" is very inclusive of anyone interested in herbalism and uses herbals to heal themselves and their families. I am certified in herbal cosmetic formulation, meaning that I am studied in the didactic and fieldwork in herbalism. This means I have studied and apprenticed cosmetics formulation in herbalism with some of the country's most renowned healers and herbalists.
We know exactly where all our raw materials/ingredients come from and their date of manufacture, and we handpick every ingredient ourselves. This ensures that the products are high quality and responsibly crafted. In addition, it gives us great autonomy and the ability to customize our formulas when needed upon retailer request or quickly pivot a formula if an ingredient becomes unavailable.
Most of our extracts are made in-house, ensuring optimal freshness and efficacy of our products. The extracts are what give a base formula its effectiveness.
All the extracts in our balms and oils are made in-house from the leaves, roots, and stems of the plants that we use in our infusions – chamomile, calendula, plantain, mullein, rose petals, arnica, green tea, hibiscus, etc. We love the color and light scent this imparts in our oils, which indicates these products are natural and true to the traditional craft of the herbal infusion practice.
This practice adds antioxidant, anti-inflammatory, and age-defying properties to our products. We are not simply purchasing premade and highly diluted extracts and isolates that are likely less than ½% of an entire formula.
Our practice gives a greater percentage of therapeutic extracts that impart full spectrum benefits and a longer shelf life. A brand can rarely do this and scale when needed to large-scale production runs as we have done.
We are a Leaping Bunny-certified brand, which requires proof that not only do we not test on animals, but all our raw ingredient vendors don't either, so our entire raw ingredient chain is Leaping Bunny-certified.
AS: In developing the Ocean Line, our first formulas were water-based (oil in water) emulsions. Binding oil and water is a scientific process that requires testing different percentages of emulsifying agents and water until you get the desired consistency. I am a perfectionist and went through about seven iterations of the Ocean Milk to get the bouncy gel (non-sticky) texture we wanted.
The good news is once we get the perfect formula, it is documented, and we make it a framework for future versions and formulations. In addition, water-based products require a preservative system that needs to be challenge-tested for months.
We are constantly consulting with chemists, scientists, and manufacturers of different natural preservatives, but no one can say definitively or promise that the chosen system will work. Working with natural preservatives is complicated because they don't contain parabens or formaldehyde, which are traditional and effective solutions. They expose customers to what may cause health issues and have even been associated with cancer.
Natural preservatives require testing different preservative "cocktails" in very small batches and then waiting to test what works and holds up over time. A preservative system that may work great for one product may not work well for every product, so unfortunately, it is not a "one size fits all" solution, and a bit of trial and error and testing (which is expensive) is part of the process. It takes time and patience.
We were lucky that every product in our ocean line tested successfully in our first round. We take this very seriously to ensure maximum safety of our products for our customers and retail clients.
It is critical to us that our products are effectively preserved and have a sound shelf life. Some natural brands market "preservative free" water-based products; however, in my opinion, I would not be comfortable releasing any water-based products that didn't have a sound preservative system in place.
AS: We are always looking to expand and hope to expand our retailer base in the coming months/years. In addition, customer acquisition for our direct-to-consumer website is an ongoing process.
As for more formulations, we are thinking of anti-aging body care. The focus always seems to be reducing facial signs of aging; however, we feel from a holistic standpoint, the body should not be ignored.
We have just launched eight new products, which is an intense and demanding process, so we will be taking a breather from formulating and focusing on marketing/sales for now.
Copyright - Unless otherwise stated all contents of this web site are © 2023 - William Reed Ltd - All Rights Reserved - Full details for the use of materials on this site can be found in the Terms & Conditions
Related topics Formulation & Science Formulation research Sustainability Trends and Emerging Markets Retail and DTC Skin Care Consumer insights Companies looking to the future
Content provided by Acme-Hardesty Company | 11-Oct-2023 | White Paper
Sharomix™ EG10, a versatile broad-spectrum antimicrobial liquid blend for preserving personal care products, ensures safety at usage levels ranging from...
Content provided by Acme-Hardesty Company | 07-Jul-2023 | Product Brochure
Acme-Hardesty’s latest expansion into Canada includes Resplanta®, Botaneco®, and BYK from our partners Sharon Personal Care and Eckart Effect Pigments...
Content provided by Covation Bio™ PDO | 22-Jun-2023 | White Paper
Zemea® propanediol is a 100% plant-based humectant and solvent that’s easy on skin and the planet. This single, natural ingredient boosts moisturization...
Content provided by Horphag Research | 10-Apr-2023 | White Paper
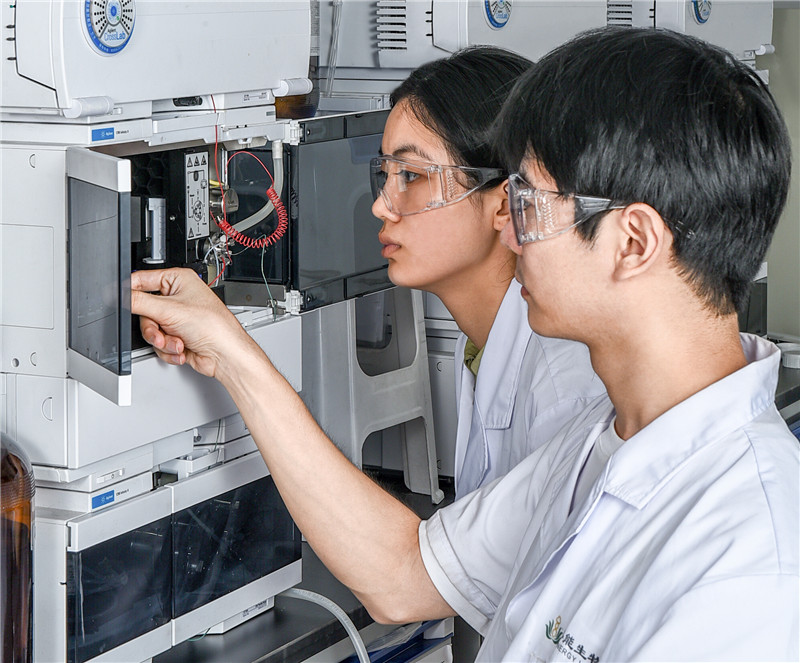
Method Of Preparation Pycnogenol® has 25+ clinical studies demonstrating its active properties for oral and topical skincare formulations. Results show the antioxidant stimulates...