Businesses need better planning to make their supply chains more agile and resilient. After explaining the shortcomings of traditional planning systems, the authors describe their new approach, optimal machine learning (OML), which has proved effective in a range of industries. A central feature is its decision-support engine that can process a vast amount of historical and current supply-and-demand data, take into account a company’s priorities, and rapidly produce recommendations for ideal production quantities, shipping arrangements, and so on. The authors explain the underpinnings of OML and provide concrete examples of how two large companies implemented it and improved their supply chains’ performance.
Flawed planning methods make it extremely difficult for companies to protect themselves against supply chain disruptions. Agricultural Machinery Wear Parts Supplier
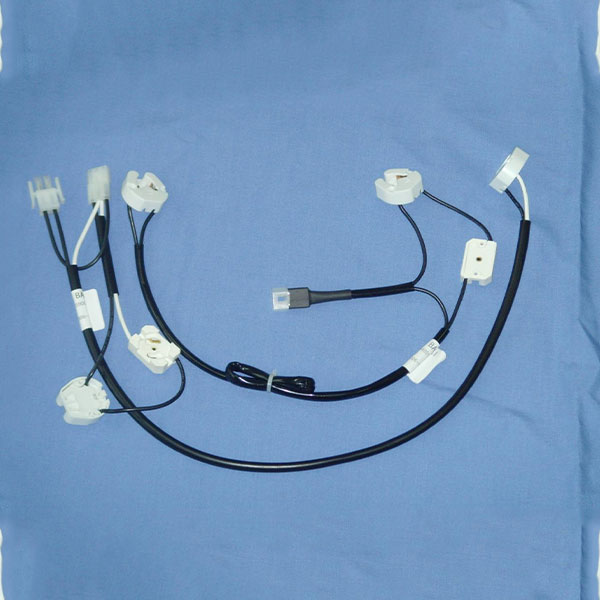
A new approach, called optimal machine learning (OML), can enable better decisions, without the mystery surrounding the planning recommendations produced by current machine-learning models.
OML relies on a decision-support engine that connects input data directly to supply chain decisions and takes into account a firm’s performance priorities. Other features are a “digital twin” representation of the entire supply chain and a data storage system that integrates information throughout the supply chain and allows for quick data access and updating.
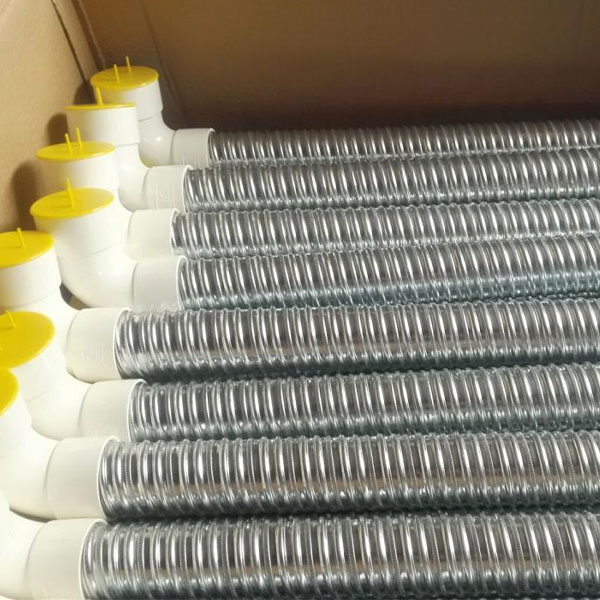
Assemblies The Covid-19 pandemic, the Russia-Ukraine conflict, trade wars, and other events in recent years have disrupted supply chains and highlighted the critical need for businesses to improve planning in order to be more agile and resilient. Yet companies struggle with this challenge. One major cause is flawed forecasting, which results in delivery delays, inventory levels that are woefully out of sync with demand, and disappointing financial performance. Those consequences are hardly surprising. After all, how can inventory and production decisions be made effectively when demand forecasts are widely off?