Light Publishing Center, Changchun Institute of Optics, Fine Mechanics And Physics, CAS
image: a, An axi-symmetric binary phase filter (BPF) and image reconstruction network are jointly learned through the physics-informed neural network. The learned BPF is fabricated and inserted in a pupil plane in an optical microscope, which produces projected volumetric image over the extended depth range. The acquired image is subsequently fed into the jointly learned reconstruction network to generate high-resolution, high-contrast image over the extended DoF. b, The viability of E2E-BPF microscope was further demonstrated by imaging a large-scale mouse kidney tissue section stained with fluorescent dyes with no refocusing (DAPI-labelled nuclei, blue; AF488-labelled tubules, green; AF568-labelled F-actin, red). OBJ: microscope objective; NA: numerical aperture; TL: tube lens; CAM: camera. view more Optics Companies

a, An axi-symmetric binary phase filter (BPF) and image reconstruction network are jointly learned through the physics-informed neural network. The learned BPF is fabricated and inserted in a pupil plane in an optical microscope, which produces projected volumetric image over the extended depth range. The acquired image is subsequently fed into the jointly learned reconstruction network to generate high-resolution, high-contrast image over the extended DoF. b, The viability of E2E-BPF microscope was further demonstrated by imaging a large-scale mouse kidney tissue section stained with fluorescent dyes with no refocusing (DAPI-labelled nuclei, blue; AF488-labelled tubules, green; AF568-labelled F-actin, red). OBJ: microscope objective; NA: numerical aperture; TL: tube lens; CAM: camera.
Credit: by Baekcheon Seong, Woovin Kim, Younghun Kim, Kyung-A Hyun, Hyo-Il Jung, Jong-Seok Lee, Jeonghoon Yoo, and Chulmin Joo
In the realm of biomedical imaging, the need for high-resolution imaging over various spatial scales in both lateral and axial dimensions is paramount. Microscopic imaging systems, which are foundational to image-based diagnosis such as cytometry, histology, and endoscopy, are often limited by the small depth-of-field (DoF). This DoF essentially determines the range over which an object can be sharply imaged, constrained by factors such as the operating wavelength and aperture size.
Conventional microscopes suffer an intrinsic trade-off between DoF and spatial resolution. High numerical-aperture objectives, while allowing for detailed visualization of cellular and subcellular features, come with limited field-of-view and DoF. As a result, the tedious process of serial refocusing at each lateral location is necessary, which is both time-consuming and labor-intensive. Though various attempts have been made over the years to enhance DoF, solutions are often not optimal, and lead to compromises in light throughput and information capacity.
In a new paper published in Light Science & Application, a team of scientists led by Professor Chulmin Joo from the Department of Mechanical Engineering at Yonsei University in Seoul, Republic of Korea, and co-workers have developed the E2E-BPF microscope, a computational imaging platform that specifically addresses these limitations. Central to its technology is the use of a binary phase filter (BPF) jointly optimized with an image reconstruction network to produce high-resolution and high-contrast images over an extended DoF, mitigating the need for refocusing.
The end-to-end framework in this study employs deep learning, jointly designing a DoF-extending BPF and the relevant imaging reconstruction network with a large dataset. Once implemented, the learned BPF enables the microscope to produce a depth-invariant point-spread function (PSF). When paired with a jointly learned deconvolution network, the E2E-BPF produces high-resolution images over 15.5 times larger DoF than a standard microscope. The scientists attribute this to an innovative design strategy for both BPF and deconvolution network:
“The adoption of such binary structure design within a deep neural network (DNN) framework poses challenges, especially with the gradient computation of binary functions. We resolved the problem by introducing a differentiable penalization function and a dedicated BPF loss term.”
“Characterized by concentric rings with binary phase states, BPFs are amenable to simple and scalable manufacturing. One of the key features of our method is the removal of restrictions on the number of concentric rings in the BPF. This not only enables a greater DoF extension but also provides additional flexibility in the BPF design, allowing it to be better optimized for specific imaging needs.”
Through a blend of numerical simulations and experiments with fluorescently labeled beads and tissue sections, the E2E-BPF microscope demonstrates its potential in high-resolution, high-contrast imaging over a DoF that is considerably larger than traditional microscopes. “This method's implications are broad, with anticipated applications in rapid image-based diagnostics and optical vision. As with all research, continued exploration and refinement, including the incorporation of system aberrations, are essential.” the scientists forecasted.
Disclaimer: AAAS and EurekAlert! are not responsible for the accuracy of news releases posted to EurekAlert! by contributing institutions or for the use of any information through the EurekAlert system.
Yaobiao Li Light Publishing Center, Changchun Institute of Optics, Fine Mechanics And Physics, CAS liyaobiao@ciomp.ac.cn Office: 86-431-861-76851
Baekcheon Seong Yonsei University, Republic of Korea bowow0310@yonsei.ac.kr
Chulmin Joo Yonsei University, Republic of Korea cjoo@yonsei.ac.kr
Light Publishing Center, Changchun Institute of Optics, Fine Mechanics And Physics, CAS
Copyright © 2024 by the American Association for the Advancement of Science (AAAS)
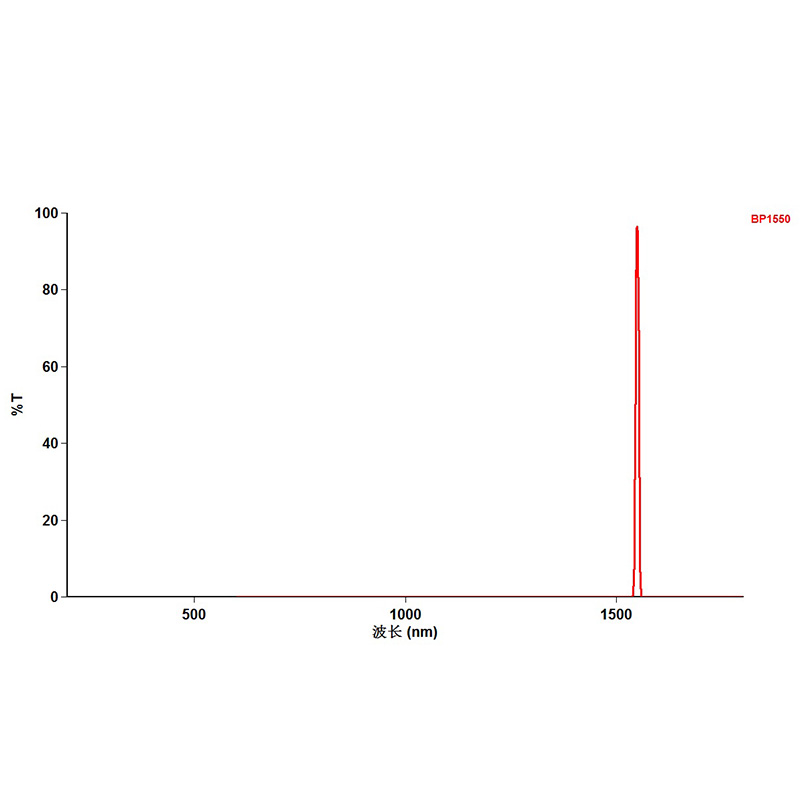
Am1.5 Filter Copyright © 2024 by the American Association for the Advancement of Science (AAAS)