Specifically, its satellite IoT (SatIoT) technology and network will be deployed within an aquaculture project in Dalatia, Croatia, to monitor water quality within remote oyster farms.
"Astrocast is excited to participate in the XGain project and support this aquaculture development in Croatia," said Fabien Jordan, CEO of Astrocast. Patient Health Monitoring System
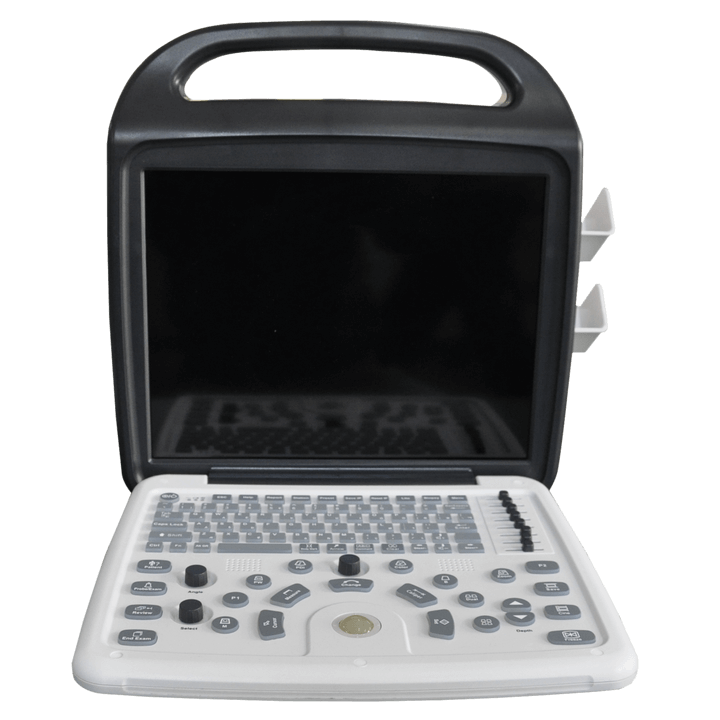
"The project focuses on providing farmers with technology tools to scale up their activities, of which connectivity is more important than ever, as it can provide them with the insights that they need about their projects, to operate them more effectively. So, we are proud to contribute with our expertise and technology to this important initiative. Especially as it aligns with our business values too."
The XGain project has been set up by the EU to facilitate the sustainable, balanced, and inclusive development of rural, coastal and urban areas with technology. It does this by providing relevant stakeholders with access to a range of smart XG, last-mile connectivity and edge computing solutions, as well as assessment methods.
At present, the XGain project comprises of a consortium of 17 partners, including Benco Baltic, which is responsible for the aquaculture project that Astrocast is participating in.
The XGain project also provides participants with a Knowledge Facilitation Tool that enables XGain to facilitate business model development for participating organisations; and provide an ecosystem of appropriate technologies.
"Benco Baltic is pleased to work with Astrocast in the aquaculture use case in Croatia," said Martynas Velicka, Benco Baltic.
"We believe the use of SatIoT technology will bring valuable insights into the water parameters of the oyster farm and help improve its performance."
In Croatia Astrocast will provide its experience in SatIoT, along with access to its communication modules, to support this aquaculture project. It will allow key water parameters to be monitored at an oyster farm near Sibenik and showcase how data can be retrieved from devices to sensors located in such remote locations.

icu heart monitor The project also demonstrates how an extended network infrastructure, supplemented by LoRaWAN and satellite communications can be used to transmit data from offshore sensors to a central monitoring system.