In probability theory, the central limit theorem (CLT) states that the distribution of a sample will approximate a normal distribution (i.e., a bell curve) as the sample size becomes larger, regardless of the population's actual distribution shape.
Put another way, CLT is a statistical premise that, given a sufficiently large sample size from a population with a finite level of variance, the mean of all sampled variables from the same population will be approximately equal to the mean of the whole population. Furthermore, these samples will approximate a normal distribution, with their variances being approximately equal to the variance of the population as the sample size gets larger, according to the law of large numbers. Cardboard Bursting Tester
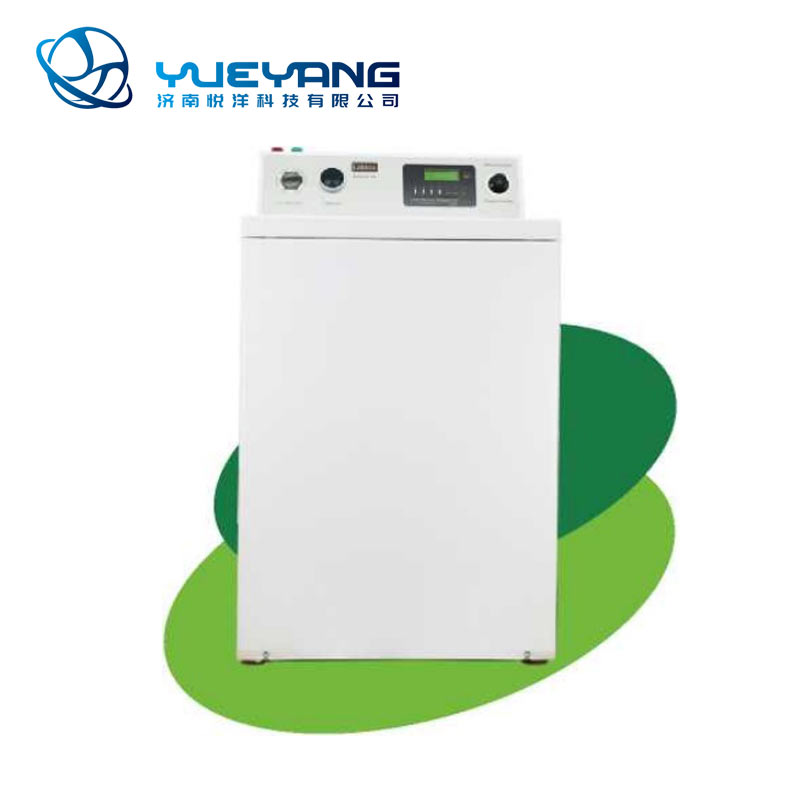
According to the central limit theorem, the mean of a sample of data will be closer to the mean of the overall population in question as the sample size increases, notwithstanding the actual distribution of the data. The concept can hold true regardless of whether the distribution of the population is normal or skewed.
As a general rule, sample sizes of 30 or more are typically deemed sufficient for the CLT to hold, meaning that the distribution of the sample means is fairly normally distributed. In addition, the more samples one takes, the more the graphed results should take the shape of a normal distribution.
The central limit theorem is often used in conjunction with the law of large numbers, which states that the average of the sample means will come closer to equaling the population mean as the sample size grows. This concept can be extremely useful in accurately predicting the characteristics of very large populations.
Although this concept was first developed by Abraham de Moivre in 1733, it was not formalized until 1920, when the Hungarian mathematician George Pólya dubbed it the central limit theorem.
The central limit theorem has several key components. They largely revolve around sampling technique.
The CLT can be helpful in examining the returns of an individual stock or of broader stock indices because the analysis is simple, due to the relative ease of generating the necessary financial data. Consequently, investors often rely on the CLT to analyze stock returns, construct portfolios, and manage risk.
The central limit theorem is useful when analyzing large data sets because it allows one to assume that the sampling distribution of the mean will be normally distributed in most cases. This allows for easier statistical analysis and inference. For example, investors can use central limit theorem to aggregate individual security performance data and generate distribution of sample means that represent a larger population distribution for security returns over a period of time.
The central limit theorem doesn't have a formula used in its practical application. Its principle is simply applied. With a sufficiently large sample size, the sample distribution will approximate a normal distribution, and the sample mean will approach the population mean. It suggests that if we have a sample size of at least 30, we can begin to analyze the data as if it fit a normal distribution.
A sample size of 30 or more is fairly common across statistics as the minimum for applying the central limit theorem. The greater your sample size, the more likely the sample will be representative of your population set.
In probability theory and statistics, the law of large numbers states that the larger the sample size, the more likely its mean is to reflect the mean of the entire population.
In business, the law of large numbers can have a different meaning, specifically that as a company grows in size, maintaining its rate of growth in percentage terms becomes more difficult.
The central limit theorem (CLT) holds that as a sample size gets larger, its mean will increasingly approximate the mean in a normal distribution. This concept can be useful in many applications, such as analyzing investment returns, because it requires only a sufficient sample size (generally interpreted as 30 or more data points) rather than the entire population.
Boston University School of Public Health. "Central Limit Theorem."
Hans Fischer. "A History of the Central Limit Theorem," Page 1.
The Mathematics Enthusiast. "Studying Moments of the Central Limit Theorem, Benjamin A. Stark."
University of Massachusetts Amherst. "What Is Central Limit Theorem? Properties, Best Practices, Examples & Everything to Know."
Emory University. "Final Summary: The Central Limit Theorem."

Pulping Papermaking Experiment Edward J. Mascha and Thomas R. Vetter. "Significance, Errors, Power, and Sample Size: The Blocking and Tackling of Statistics." Anesthesia & Analgesia. 2018 Feb;126(2):691-698.